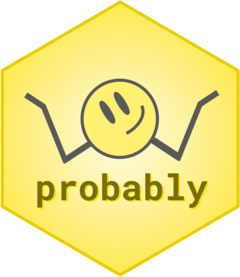
Measure performance with and without using bagged isotonic regression calibration
Source:R/cal-validate.R
cal_validate_isotonic_boot.Rd
This function uses resampling to measure the effect of calibrating predicted values.
Usage
cal_validate_isotonic_boot(
.data,
truth = NULL,
estimate = dplyr::starts_with(".pred"),
metrics = NULL,
save_pred = FALSE,
...
)
# S3 method for class 'resample_results'
cal_validate_isotonic_boot(
.data,
truth = NULL,
estimate = dplyr::starts_with(".pred"),
metrics = NULL,
save_pred = FALSE,
...
)
# S3 method for class 'rset'
cal_validate_isotonic_boot(
.data,
truth = NULL,
estimate = dplyr::starts_with(".pred"),
metrics = NULL,
save_pred = FALSE,
...
)
# S3 method for class 'tune_results'
cal_validate_isotonic_boot(
.data,
truth = NULL,
estimate = NULL,
metrics = NULL,
save_pred = FALSE,
...
)
Arguments
- .data
An
rset
object or the results oftune::fit_resamples()
with a.predictions
column.- truth
The column identifier for the true class results (that is a factor). This should be an unquoted column name.
- estimate
A vector of column identifiers, or one of
dplyr
selector functions to choose which variables contains the class probabilities. It defaults to the prefix used by tidymodels (.pred_
). The order of the identifiers will be considered the same as the order of the levels of thetruth
variable.- metrics
A set of metrics passed created via
yardstick::metric_set()
- save_pred
Indicates whether to a column of post-calibration predictions.
- ...
Options to pass to
cal_estimate_isotonic_boot()
, such as thetimes
argument.
Value
The original object with a .metrics_cal
column and, optionally,
an additional .predictions_cal
column. The class cal_rset
is also added.
Details
These functions are designed to calculate performance with and without calibration. They use resampling to measure out-of-sample effectiveness. There are two ways to pass the data in:
If you have a data frame of predictions, an
rset
object can be created via rsample functions. See the example below.If you have already made a resampling object from the original data and used it with
tune::fit_resamples()
, you can pass that object to the calibration function and it will use the same resampling scheme. If a different resampling scheme should be used, runtune::collect_predictions()
on the object and use the process in the previous bullet point.
Please note that these functions do not apply to tune_result
objects. The
notion of "validation" implies that the tuning parameter selection has been
resolved.
collect_predictions()
can be used to aggregate the metrics for analysis.
Performance Metrics
By default, the average of the Brier scores (classification calibration) or the
root mean squared error (regression) is returned. Any appropriate
yardstick::metric_set()
can be used. The validation function compares the
average of the metrics before, and after the calibration.
Examples
library(dplyr)
segment_logistic |>
rsample::vfold_cv() |>
cal_validate_isotonic_boot(Class)
#> # 10-fold cross-validation
#> # A tibble: 10 × 4
#> splits id .metrics .metrics_cal
#> <list> <chr> <list> <list>
#> 1 <split [909/101]> Fold01 <tibble [1 × 3]> <tibble [1 × 3]>
#> 2 <split [909/101]> Fold02 <tibble [1 × 3]> <tibble [1 × 3]>
#> 3 <split [909/101]> Fold03 <tibble [1 × 3]> <tibble [1 × 3]>
#> 4 <split [909/101]> Fold04 <tibble [1 × 3]> <tibble [1 × 3]>
#> 5 <split [909/101]> Fold05 <tibble [1 × 3]> <tibble [1 × 3]>
#> 6 <split [909/101]> Fold06 <tibble [1 × 3]> <tibble [1 × 3]>
#> 7 <split [909/101]> Fold07 <tibble [1 × 3]> <tibble [1 × 3]>
#> 8 <split [909/101]> Fold08 <tibble [1 × 3]> <tibble [1 × 3]>
#> 9 <split [909/101]> Fold09 <tibble [1 × 3]> <tibble [1 × 3]>
#> 10 <split [909/101]> Fold10 <tibble [1 × 3]> <tibble [1 × 3]>